Automate text extraction from images using GCP Vision and Python
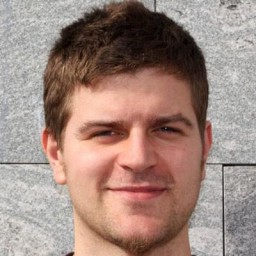
In today's digital landscape, automating text extraction from images and documents is crucial for data processing, archival, and analysis. Optical Character Recognition (OCR) using Google Cloud Vision API provides a powerful solution for converting physical documents into searchable digital formats. This guide demonstrates how to implement OCR with Python for efficient document digitization.
Requirements
- Python 3.7+
- Google Cloud Platform account
- Basic Python knowledge
Set up Google cloud environment
- Create a project in Google Cloud Console
- Enable the Cloud Vision API
- Configure authentication:
Development setup
gcloud auth application-default login
gcloud auth application-default set-quota-project PROJECT_ID
Production authentication
- Create service account and download JSON key
- Set environment variable:
export GOOGLE_APPLICATION_CREDENTIALS="path/to/service-account-key.json"
Configure Python environment
- Create virtual environment:
python3 -m venv venv
source venv/bin/activate # Linux/macOS
venv\Scripts\activate # Windows
- Install client library:
pip install google-cloud-vision
Implement OCR solution
Robust implementation with error handling and text post-processing:
from google.cloud import vision
from google.api_core import retry
import io
import sys
def extract_text(image_path: str) -> str:
"""Extracts text from an image using GCP Vision API.
Args:
image_path: Path to image file (JPEG, PNG, PDF)
Returns:
Extracted text as single string
Raises:
Exception: API errors or file processing issues
"""
client = vision.ImageAnnotatorClient()
try:
with io.open(image_path, 'rb') as image_file:
content = image_file.read()
image = vision.Image(content=content)
response = client.document_text_detection(
image=image,
retry=retry.Retry(initial=1.0, maximum=10.0)
)
if response.error.message:
raise RuntimeError(f'API Error: {response.error.message}')
return response.full_text_annotation.text
except Exception as e:
print(f'OCR processing failed: {str(e)}')
raise
if __name__ == '__main__':
if len(sys.argv) != 2:
print('Usage: python extract_text.py <image_path>')
sys.exit(1)
try:
print(extract_text(sys.argv[1]))
except Exception as e:
print(f'Error: {str(e)}')
sys.exit(1)
Production considerations
- File Formats: Supports JPEG, PNG, PDF, and TIFF
- PDF Limits: Up to 2000 pages per document
- Rate Limits: 1800 requests/minute default quota
- Cost Optimization: Use async processing for batch operations
- Text Localization: Handles 100+ languages automatically
Advanced processing techniques
Enhance OCR results with text normalization and structure extraction:
def process_ocr_text(raw_text: str) -> dict:
"""Structure raw OCR output into organized data"""
return {
'full_text': raw_text,
'paragraphs': raw_text.split('\n\n'),
'line_count': len(raw_text.split('\n')),
'word_count': len(raw_text.split()),
'cleaned_text': raw_text.replace('\n', ' ').strip()
}
Error handling improvements
Implement retry logic and input validation:
from google.api_core import retry
import logging
logger = logging.getLogger(__name__)
@retry.Retry(
predicate=retry.if_exception_type(
ResourceExhausted,
ServiceUnavailable
)
)
def safe_ocr_call(image: vision.Image) -> vision.TextAnnotation:
"""Wrapper with enhanced retry configuration"""
return client.document_text_detection(image=image)
Conclusion
Implementing OCR with Google Cloud Vision API and Python provides a scalable solution for document digitization. The techniques shown here handle various document types while maintaining production reliability. For advanced document processing workflows requiring PDF manipulation or multi-file operations, consider exploring Transloadit's document processing solutions.
Happy coding!